The Ultimate Guide to Image Labeling Tools for Object Detection
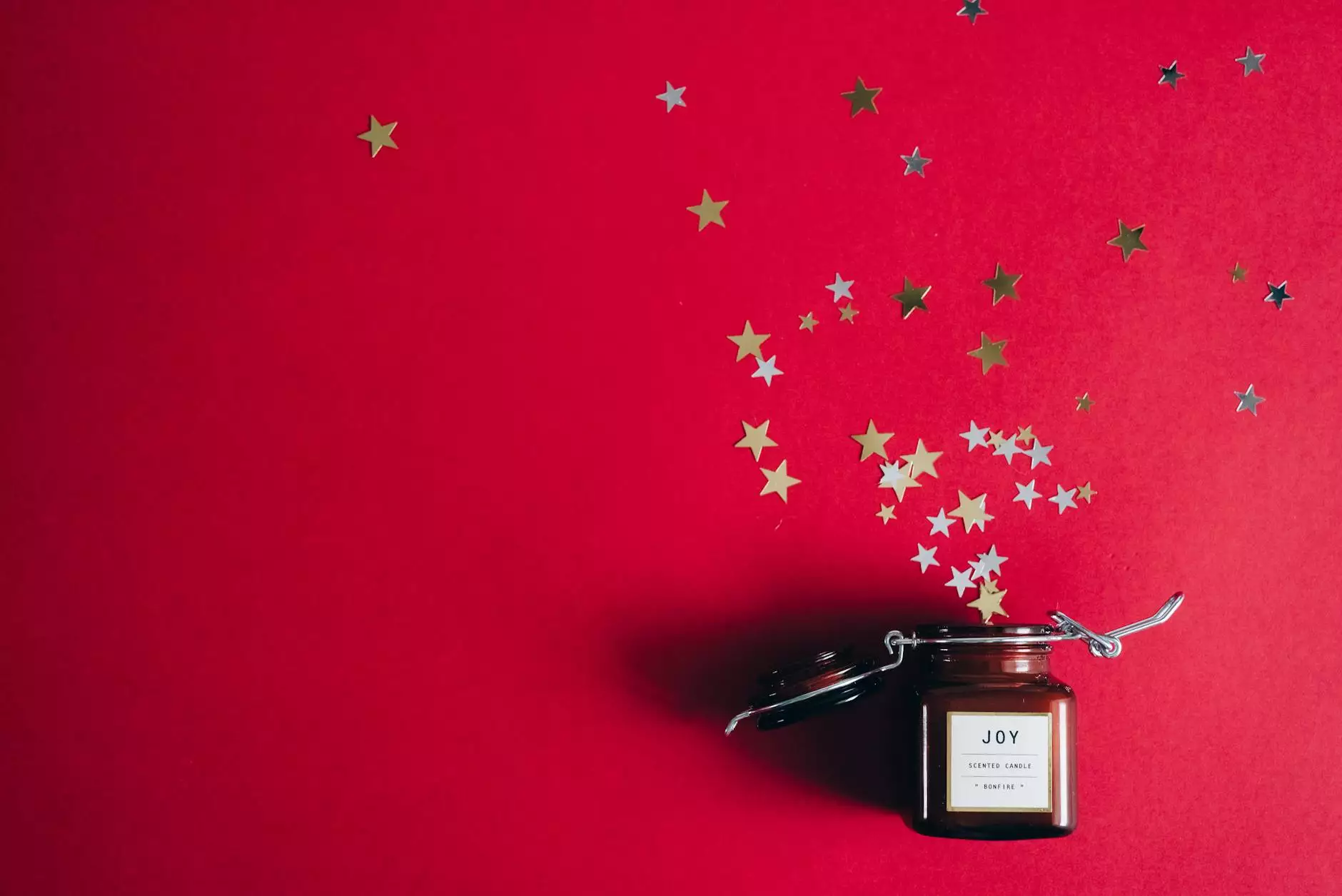
In today's world, the utilization of artificial intelligence (AI) and machine learning (ML) in various industries has become increasingly prevalent. At the heart of this technological revolution lies an essential process known as data annotation. This process is particularly critical in developing robust AI models for tasks like object recognition and detection. One of the cornerstones of effective data annotation is the image labeling tool for object detection. In this comprehensive guide, we will explore the significance of these tools, their functionalities, best practices, and their vital role in the broader context of data annotation.
Understanding Image Labeling and Its Importance
Image labeling refers to the process of tagging images with descriptive labels that define the different objects present within them. This procedure enables machine learning models to recognize and understand various objects in a visual context. The significance of accurate labeling cannot be overstated, as it directly influences the model's capability to identify and classify objects effectively.
The Role of Image Labeling Tools
Image labeling tools are software solutions designed to facilitate and streamline the image annotation process. These tools provide users with the functionality to annotate images efficiently, ensuring high-quality output that is vital for training machine learning models. Utilizing advanced algorithms and powerful user interfaces, these tools can significantly enhance productivity and accuracy in labeling.
The Process of Object Detection
Object detection is a sub-field of computer vision aimed at detecting instances of objects within images or videos. It incorporates both image classification to identify the object type and object localization to pinpoint where the object is located within the frame. The object detection process entails the following steps:
- Data Collection: Gathering a diverse set of images for annotation.
- Image Annotation: Using image labeling tools to accurately tag the images.
- Model Training: Feeding annotated data into machine learning models.
- Evaluation: Testing the models on unseen data to evaluate performance.
- Refinement: Iterating on the model based on evaluation metrics.
Types of Image Labeling Tools
There are several types of image labeling tools for object detection, each serving different needs and objectives. Here are some of the most commonly used tools:
1. Manual Annotation Tools
These tools require human annotators to draw bounding boxes, polygons, or other shapes around objects in images. They are ideal for projects that require high precision and specific labeling:
- LabelImg
- VoTT (Visual Object Tagging Tool)
2. Semi-Automatic Annotation Tools
These tools offer automated suggestions based on pre-trained models, allowing annotators to quickly adjust and correct the labels:
- SuperAnnotate
- RectLabel
3. Fully Automated Annotation Tools
These sophisticated tools utilize AI algorithms to automatically detect and label objects in images. While they may require initial training data, they significantly reduce human workload:
- TensorFlow Object Detection API
- Cloud-based annotation services like Keylabs.ai
Key Features to Consider in an Image Labeling Tool
When selecting an image labeling tool for object detection, various features should be considered to ensure it meets your project needs. Here are some essential features:1. User-Friendly Interface
A tool with an intuitive user interface will facilitate easier navigation and quicker onboarding for new users, saving time in the annotation process.
2. Support for Multiple Formats
Your chosen tool should support various image formats and annotations, ensuring compatibility with different machine learning frameworks and workflows.
3. Collaborative Features
If your project involves multiple annotators or teams, look for tools that offer collaborative features to streamline communication and workflow.
4. Quality Control Mechanisms
Implementing quality assurance measures such as validation checks can help maintain the integrity of the annotations.
5. Integration Capabilities
The tool should easily integrate with existing machine learning frameworks, enabling a smoother transition from annotation to model training.
The Benefits of Using an Image Labeling Tool for Object Detection
Utilizing an image labeling tool offers numerous advantages:1. Increased Efficiency
Automation and streamlined processes significantly speed up the annotation workflow, allowing teams to focus on model development rather than laborious manual tasks.
2. Improved Accuracy
High-quality tools often include features that help minimize human error, ensuring more precise labels, which contribute to better model performance.
3. Cost-Effective Solutions
By reducing the time and resources spent on data annotation, businesses can allocate budgets towards other critical areas, enhancing overall productivity.
Best Practices for Using Image Labeling Tools
To maximize the effectiveness of your image labeling tool for object detection, consider the following best practices:
1. Define Clear Annotation Guidelines
Establishing clear guidelines helps maintain consistency across your data annotations. Provide annotators with detailed instructions and examples of the desired outcomes.
2. Regularly Evaluate and Audit Annotations
Conduct regular evaluations to identify and correct any inconsistencies or errors in the annotations, ensuring the quality of the training data is maintained.
3. Leverage Automation Wisely
While automation tools can boost efficiency, they should be used to supplement human efforts, not replace them. Always have a human in the loop to verify and make necessary adjustments.
4. Choose the Right Tool for Your Needs
Assess your specific requirements to select the most suitable tool, whether you need manual, semi-automated, or fully automated capabilities.
Conclusion: Harnessing the Power of Image Labeling Tools
In conclusion, the landscape of machine learning and artificial intelligence is ever-evolving, and the role of data annotation, specifically through the use of image labeling tools for object detection, is paramount. As businesses and sectors across the globe harness these technologies, the demand for high-quality labeled data continues to rise. By understanding the importance of these tools, their functionalities, and the best practices for their usage, organizations can stay ahead of the curve in developing intelligent, responsive AI models.
For businesses looking to explore comprehensive and effective solutions in the realm of data annotation, platforms like Keylabs.ai offer exceptional user-friendly interfaces and powerful features tailored to meet the evolving needs of modern enterprises. Invest in the right image labeling tool today, and elevate your AI initiatives to new heights!